Unplanned downtime poses a significant financial burden, with costs averaging around $125,000 per hour across various industries. This challenge is particularly pronounced in sectors such as oil and gas, chemicals, and metals, where critical outages can occur multiple times a year.
To mitigate these costly disruptions, investing in predictive maintenance (PdM) has emerged as a viable solution, offering the potential for substantial savings through timely interventions. In the fast-paced landscape of modern industry, ensuring equipment reliability is paramount.
Unexpected downtime can lead to lost productivity, expensive repairs, and safety hazards. Predictive maintenance technologies, a cornerstone of Industry 4.0, are designed to address these challenges by predicting equipment failures before they occur.
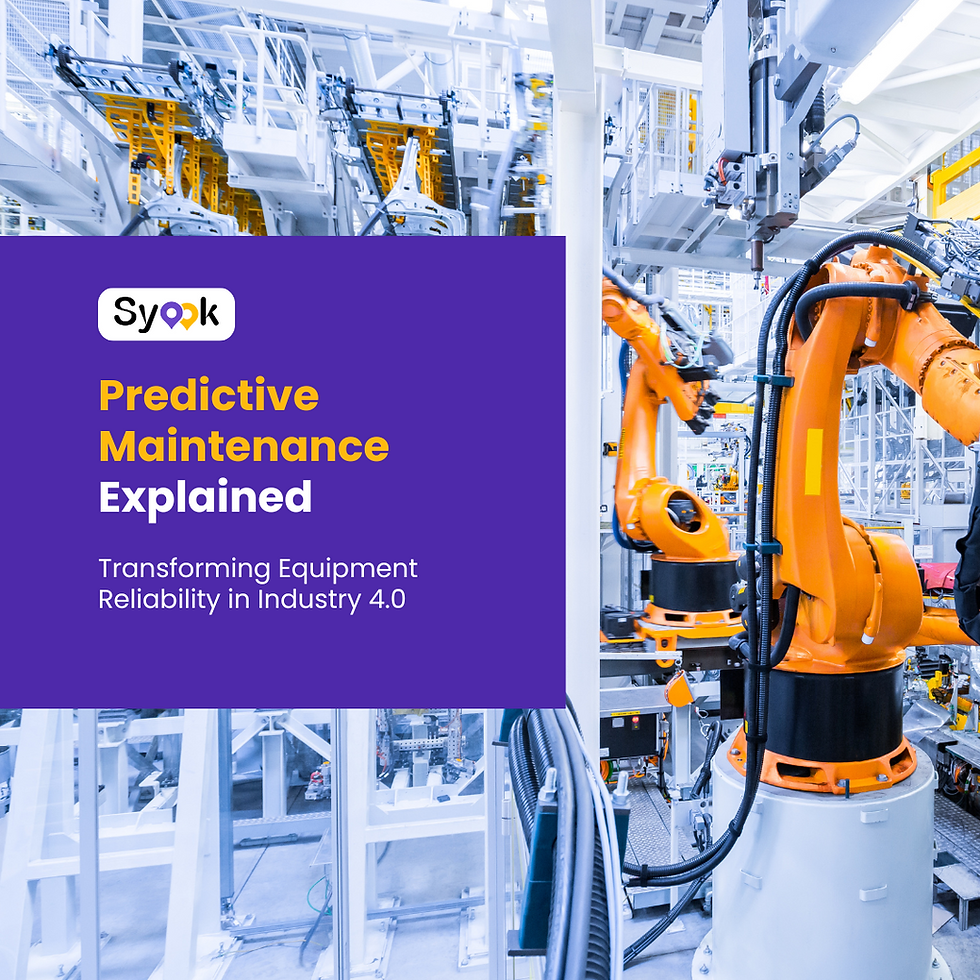
What is Predictive Maintenance?
Predictive maintenance represents the pinnacle of maintenance strategies, utilizing data-driven insights to forecast potential equipment failures. By analyzing real-time data from IoT sensors and employing machine learning algorithms, PdM enables businesses to schedule maintenance only when necessary, thereby reducing unnecessary downtime and extending the lifespan of machinery.
The Evolution of Maintenance Strategies
The journey to predictive maintenance can be categorized into four phases:
Maintenance 1.0: Reactive maintenance focused solely on fixing equipment after failures occurred.
Maintenance 2.0: Preventive maintenance introduced scheduled checks to reduce breakdowns but often resulted in unnecessary tasks.
Maintenance 3.0: Condition-based maintenance utilized sensor data to inform maintenance decisions based on actual equipment conditions.
Maintenance 4.0: The current phase leverages IoT, AI, and big data analytics to predict when equipment might fail.
How Predictive Maintenance Works?
The predictive maintenance process involves several key steps:
Data Collection: IoT sensors continuously gather data on various parameters such as vibration and temperature.
Data Analysis: Advanced algorithms analyze this data to identify patterns and potential issues.
Prediction and Alert: The system generates alerts for the maintenance team when anomalies are detected.
Continuous Improvement: As more data is collected, the accuracy of predictions improves over time.
Types of Predictive Maintenance
Three primary types of predictive maintenance have emerged:
Indirect Failure Prediction: Uses machine health scores based on historical data; scalable but less precise.
Anomaly Detection: Flags deviations from normal behaviour; lower data requirements but potential for false positives.
Remaining Useful Life (RUL) Estimation: Provides precise predictions on remaining operational time; highly accurate but resource-intensive.
Challenges in Implementing Predictive Maintenance
Despite its advantages, implementing predictive maintenance presents challenges:
High Startup Costs: Initial investments in technology can be substantial.
Complexity: Integrating systems and managing large datasets can be complicated.
Data Quality: Effective predictive maintenance relies on high-quality data from sensors.
Over-Reliance on Technology: Balancing technology with human expertise is crucial for successful implementation.
Industry Applications
Predictive maintenance is gaining traction across various sectors, here are a few examples
Manufacturing: Minimizes production line disruptions through effective monitoring.
Energy: Prevents outages by maintaining critical infrastructure health.
Transportation: Ensures timely vehicle maintenance to reduce breakdown risks.
Oil and Gas: Monitors infrastructure health to prevent costly disruptions.
Future Trends in Predictive Maintenance
The future of predictive maintenance is bright, driven by advancements in technology:
IoT and Big Data: Enhanced data generation improves prediction accuracy.
Digital Twins: Virtual replicas allow for simulation and outcome prediction without risking actual equipment.
Predictive Maintenance as a Service (PMaaS): Offers accessible solutions without hefty upfront investments.
Integration with CMMS/EAM Platforms: Streamlines processes and enhances decision-making capabilities.
Conclusion
Predictive maintenance signifies a transformative approach to managing equipment health within industries. By leveraging IoT, condition monitoring, and advanced analytics, PdM provides a proactive strategy that not only reduces downtime but also extends equipment lifespan and enhances safety.
As Industry 4.0 technologies continue to evolve, predictive maintenance will become increasingly vital for maintaining competitive advantages across various sectors.Unlock the full potential of your
Comments